TR2012-012
Greedy Sparsity-Constrained Optimization
-
- "Greedy Sparsity-Constrained Optimization", Asilomar Conference on Signals, Systems and Computers (ACSSC), DOI: 10.1109/ACSSC.2011.6190194, November 2011, pp. 1148-1152.BibTeX TR2012-012 PDF
- @inproceedings{Bahmani2011nov,
- author = {Bahmani, S. and Boufounos, P. and Raj, B.},
- title = {{Greedy Sparsity-Constrained Optimization}},
- booktitle = {Asilomar Conference on Signals, Systems and Computers (ACSSC)},
- year = 2011,
- pages = {1148--1152},
- month = nov,
- doi = {10.1109/ACSSC.2011.6190194},
- url = {https://www.merl.com/publications/TR2012-012}
- }
,
- "Greedy Sparsity-Constrained Optimization", Asilomar Conference on Signals, Systems and Computers (ACSSC), DOI: 10.1109/ACSSC.2011.6190194, November 2011, pp. 1148-1152.
-
MERL Contact:
-
Research Area:
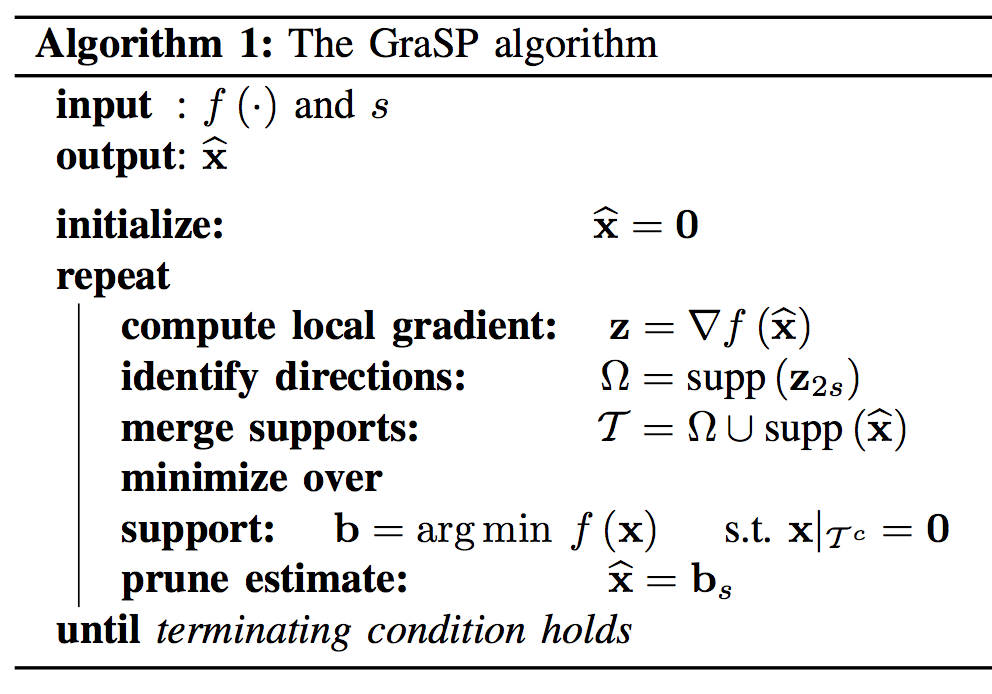
Abstract:
Finding optimal sparse solutions to estimation problems, particularly in under-determined regimes has recently gained much attention. Most existing literature study linear models in which the squared error is used as the measure of discrepancy to be minimized. However, in many applications discrepancy is measured in more general forms such as log-likelihood. Regularization by '1-norm has been shown to induce sparse solutions, but their sparsity level can be merely suboptimal. In this paper we present a greedy algorithm, dubbed Gradient Support Pursuit (GraSP), for sparsity constrained optimization. Quantifiable guarantees are provided for GraSP when cost functions have the Stable Hessian Property.
Related News & Events
-
NEWS ACSSC 2011: publication by Petros T. Boufounos and others Date: November 6, 2011
Where: Asilomar Conference on Signals, Systems and Computers (ACSSC)
MERL Contact: Petros T. Boufounos
Research Area: Computational SensingBrief- The paper "Greedy Sparsity-Constrained Optimization" by Bahmani, S., Boufounos, P. and Raj, B. was presented at the Asilomar Conference on Signals, Systems and Computers (ACSSC).
Related Publication
BibTeX TR2013-015 PDF
- @article{Bahmani2013mar,
- author = {Bahmani, S. and Raj, B. and Boufounos, P.},
- title = {{Greedy Sparsity-Constrained Optimization}},
- journal = {Journal of Machine Learning Research (JMLR)},
- year = 2013,
- volume = 14,
- pages = {807--841},
- month = mar,
- url = {https://www.merl.com/publications/TR2013-015}
- }