TR2014-022
Deep recurrent de-noising auto-encoder and blind de-reverberation for reverberated speech recognition
-
- "Deep Recurrent De-noising Auto-encoder and Blind De-reverberation for Reverberated Speech Recognition", IEEE International Conference on Acoustics, Speech, and Signal Processing (ICASSP), DOI: 10.1109/ICASSP.2014.6854478, May 2014, pp. 4623-4627.BibTeX TR2014-022 PDF
- @inproceedings{Weninger2014may1,
- author = {Weninger, F. and Watanabe, S. and Tachioka, Y. and Schuller, B.},
- title = {{Deep Recurrent De-noising Auto-encoder and Blind De-reverberation for Reverberated Speech Recognition}},
- booktitle = {IEEE International Conference on Acoustics, Speech, and Signal Processing (ICASSP)},
- year = 2014,
- pages = {4623--4627},
- month = may,
- publisher = {IEEE},
- doi = {10.1109/ICASSP.2014.6854478},
- url = {https://www.merl.com/publications/TR2014-022}
- }
,
- "Deep Recurrent De-noising Auto-encoder and Blind De-reverberation for Reverberated Speech Recognition", IEEE International Conference on Acoustics, Speech, and Signal Processing (ICASSP), DOI: 10.1109/ICASSP.2014.6854478, May 2014, pp. 4623-4627.
-
Research Areas:
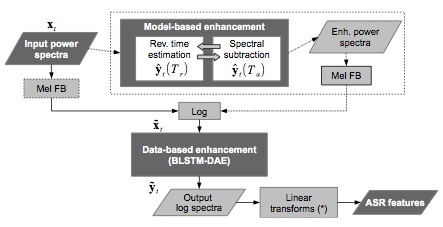
Abstract:
This paper describes our joint efforts to provide robust automatic speech recognition (ASR) for reverberated environments, such as in hands-free human-machine interaction. We investigate blind feature space de-reverberation and deep recurrent de-noising auto-encoders (DAE) in an early fusion scheme. Results on the 2014 REVERB Challenge development set indicate that the DAE front-end provides complementary performance gains to multi-condition training, feature transformations, and model adaptation. The proposed ASR system achieves word error rates of 17.62 % and 36.6 % on simulated and real data, which is a significant improvement over the Challenge baseline (25.16 and 47.2 %).