Moitreya Chatterjee
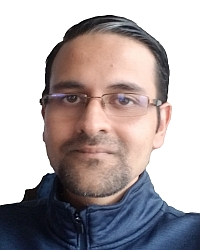
- Phone: 617-621-7592
- Email:
-
Position:
Research / Technical Staff
Research Scientist -
Education:
Ph.D., University of Illinois at Urbana-Champaign, 2022 -
Research Areas:
Moitreya's Quick Links
-
Biography
Moitreya's research interests are in computer vision, and multimodal machine learning with a particular emphasis on learning from audio-visual data. His PhD work received the Joan and Lalit Bahl Fellowship and the Thomas and Margaret Huang Research Award. Earlier, he earned a M.S. degree in Computer Science from the University of Southern California (USC), during which he received an Outstanding Paper Award from the ACM International Conference on Multimodal Interaction (ICMI).
-
Recent News & Events
-
NEWS MERL Papers and Workshops at CVPR 2025 Date: June 11, 2025 - June 15, 2025
Where: Nashville, TN, USA
MERL Contacts: Matthew Brand; Moitreya Chatterjee; Anoop Cherian; François Germain; Michael J. Jones; Toshiaki Koike-Akino; Jing Liu; Suhas Lohit; Tim K. Marks; Pedro Miraldo; Kuan-Chuan Peng; Pu (Perry) Wang; Ye Wang
Research Areas: Artificial Intelligence, Computer Vision, Machine Learning, Signal Processing, Speech & AudioBrief- MERL researchers are presenting 2 conference papers, co-organizing two workshops, and presenting 7 workshop papers at the IEEE Conference on Computer Vision and Pattern Recognition (CVPR) 2025 conference, which will be held in Nashville, TN, USA from June 11-15, 2025. CVPR is one of the most prestigious and competitive international conferences in the area of computer vision. Details of MERL contributions are provided below:
Main Conference Papers:
1. "UWAV: Uncertainty-weighted Weakly-supervised Audio-Visual Video Parsing" by Y.H. Lai, J. Ebbers, Y. F. Wang, F. Germain, M. J. Jones, M. Chatterjee
This work deals with the task of weakly‑supervised Audio-Visual Video Parsing (AVVP) and proposes a novel, uncertainty-aware algorithm called UWAV towards that end. UWAV works by producing more reliable segment‑level pseudo‑labels while explicitly weighting each label by its prediction uncertainty. This uncertainty‑aware training, combined with a feature‑mixup regularization scheme, promotes inter‑segment consistency in the pseudo-labels. As a result, UWAV achieves state‑of‑the‑art performance on two AVVP datasets across multiple metrics, demonstrating both effectiveness and strong generalizability.
Paper: https://www.merl.com/publications/TR2025-072
2. "TailedCore: Few-Shot Sampling for Unsupervised Long-Tail Noisy Anomaly Detection" by Y. G. Jung, J. Park, J. Yoon, K.-C. Peng, W. Kim, A. B. J. Teoh, and O. Camps.
This work tackles unsupervised anomaly detection in complex scenarios where normal data is noisy and has an unknown, imbalanced class distribution. Existing models face a trade-off between robustness to noise and performance on rare (tail) classes. To address this, the authors propose TailSampler, which estimates class sizes from embedding similarities to isolate tail samples. Using TailSampler, they develop TailedCore, a memory-based model that effectively captures tail class features while remaining noise-robust, outperforming state-of-the-art methods in extensive evaluations.
paper: https://www.merl.com/publications/TR2025-077
MERL Co-Organized Workshops:
1. Multimodal Algorithmic Reasoning (MAR) Workshop, organized by A. Cherian, K.-C. Peng, S. Lohit, H. Zhou, K. Smith, L. Xue, T. K. Marks, and J. Tenenbaum.
Workshop link: https://marworkshop.github.io/cvpr25/
2. The 6th Workshop on Fair, Data-Efficient, and Trusted Computer Vision, organized by N. Ratha, S. Karanam, Z. Wu, M. Vatsa, R. Singh, K.-C. Peng, M. Merler, and K. Varshney.
Workshop link: https://fadetrcv.github.io/2025/
Workshop Papers:
1. "FreBIS: Frequency-Based Stratification for Neural Implicit Surface Representations" by N. Sawada, P. Miraldo, S. Lohit, T.K. Marks, and M. Chatterjee (Oral)
With their ability to model object surfaces in a scene as a continuous function, neural implicit surface reconstruction methods have made remarkable strides recently, especially over classical 3D surface reconstruction methods, such as those that use voxels or point clouds. Towards this end, we propose FreBIS - a neural implicit‑surface framework that avoids overloading a single encoder with every surface detail. It divides a scene into several frequency bands and assigns a dedicated encoder (or group of encoders) to each band, then enforces complementary feature learning through a redundancy‑aware weighting module. Swapping this frequency‑stratified stack into an off‑the‑shelf reconstruction pipeline markedly boosts 3D surface accuracy and view‑consistent rendering on the challenging BlendedMVS dataset.
paper: https://www.merl.com/publications/TR2025-074
2. "Multimodal 3D Object Detection on Unseen Domains" by D. Hegde, S. Lohit, K.-C. Peng, M. J. Jones, and V. M. Patel.
LiDAR-based object detection models often suffer performance drops when deployed in unseen environments due to biases in data properties like point density and object size. Unlike domain adaptation methods that rely on access to target data, this work tackles the more realistic setting of domain generalization without test-time samples. We propose CLIX3D, a multimodal framework that uses both LiDAR and image data along with supervised contrastive learning to align same-class features across domains and improve robustness. CLIX3D achieves state-of-the-art performance across various domain shifts in 3D object detection.
paper: https://www.merl.com/publications/TR2025-078
3. "Improving Open-World Object Localization by Discovering Background" by A. Singh, M. J. Jones, K.-C. Peng, M. Chatterjee, A. Cherian, and E. Learned-Miller.
This work tackles open-world object localization, aiming to detect both seen and unseen object classes using limited labeled training data. While prior methods focus on object characterization, this approach introduces background information to improve objectness learning. The proposed framework identifies low-information, non-discriminative image regions as background and trains the model to avoid generating object proposals there. Experiments on standard benchmarks show that this method significantly outperforms previous state-of-the-art approaches.
paper: https://www.merl.com/publications/TR2025-058
4. "PF3Det: A Prompted Foundation Feature Assisted Visual LiDAR 3D Detector" by K. Li, T. Zhang, K.-C. Peng, and G. Wang.
This work addresses challenges in 3D object detection for autonomous driving by improving the fusion of LiDAR and camera data, which is often hindered by domain gaps and limited labeled data. Leveraging advances in foundation models and prompt engineering, the authors propose PF3Det, a multi-modal detector that uses foundation model encoders and soft prompts to enhance feature fusion. PF3Det achieves strong performance even with limited training data. It sets new state-of-the-art results on the nuScenes dataset, improving NDS by 1.19% and mAP by 2.42%.
paper: https://www.merl.com/publications/TR2025-076
5. "Noise Consistency Regularization for Improved Subject-Driven Image Synthesis" by Y. Ni., S. Wen, P. Konius, A. Cherian
Fine-tuning Stable Diffusion enables subject-driven image synthesis by adapting the model to generate images containing specific subjects. However, existing fine-tuning methods suffer from two key issues: underfitting, where the model fails to reliably capture subject identity, and overfitting, where it memorizes the subject image and reduces background diversity. To address these challenges, two auxiliary consistency losses are porposed for diffusion fine-tuning. First, a prior consistency regularization loss ensures that the predicted diffusion noise for prior (non- subject) images remains consistent with that of the pretrained model, improving fidelity. Second, a subject consistency regularization loss enhances the fine-tuned model’s robustness to multiplicative noise modulated latent code, helping to preserve subject identity while improving diversity. Our experimental results demonstrate the effectiveness of our approach in terms of image diversity, outperforming DreamBooth in terms of CLIP scores, background variation, and overall visual quality.
paper: https://www.merl.com/publications/TR2025-073
6. "LatentLLM: Attention-Aware Joint Tensor Compression" by T. Koike-Akino, X. Chen, J. Liu, Y. Wang, P. Wang, M. Brand
We propose a new framework to convert a large foundation model such as large language models (LLMs)/large multi- modal models (LMMs) into a reduced-dimension latent structure. Our method uses a global attention-aware joint tensor decomposition to significantly improve the model efficiency. We show the benefit on several benchmark including multi-modal reasoning tasks.
paper: https://www.merl.com/publications/TR2025-075
7. "TuneComp: Joint Fine-Tuning and Compression for Large Foundation Models" by T. Koike-Akino, X. Chen, J. Liu, Y. Wang, P. Wang, M. Brand
To reduce model size during post-training, compression methods, including knowledge distillation, low-rank approximation, and pruning, are often applied after fine- tuning the model. However, sequential fine-tuning and compression sacrifices performance, while creating a larger than necessary model as an intermediate step. In this work, we aim to reduce this gap, by directly constructing a smaller model while guided by the downstream task. We propose to jointly fine-tune and compress the model by gradually distilling it to a pruned low-rank structure. Experiments demonstrate that joint fine-tuning and compression significantly outperforms other sequential compression methods.
paper: https://www.merl.com/publications/TR2025-079
- MERL researchers are presenting 2 conference papers, co-organizing two workshops, and presenting 7 workshop papers at the IEEE Conference on Computer Vision and Pattern Recognition (CVPR) 2025 conference, which will be held in Nashville, TN, USA from June 11-15, 2025. CVPR is one of the most prestigious and competitive international conferences in the area of computer vision. Details of MERL contributions are provided below:
-
NEWS MERL Papers and Workshops at CVPR 2024 Date: June 17, 2024 - June 21, 2024
Where: Seattle, WA
MERL Contacts: Petros T. Boufounos; Moitreya Chatterjee; Anoop Cherian; Michael J. Jones; Toshiaki Koike-Akino; Jonathan Le Roux; Suhas Lohit; Tim K. Marks; Pedro Miraldo; Jing Liu; Kuan-Chuan Peng; Pu (Perry) Wang; Ye Wang; Matthew Brand
Research Areas: Artificial Intelligence, Computational Sensing, Computer Vision, Machine Learning, Speech & AudioBrief- MERL researchers are presenting 5 conference papers, 3 workshop papers, and are co-organizing two workshops at the CVPR 2024 conference, which will be held in Seattle, June 17-21. CVPR is one of the most prestigious and competitive international conferences in computer vision. Details of MERL contributions are provided below.
CVPR Conference Papers:
1. "TI2V-Zero: Zero-Shot Image Conditioning for Text-to-Video Diffusion Models" by H. Ni, B. Egger, S. Lohit, A. Cherian, Y. Wang, T. Koike-Akino, S. X. Huang, and T. K. Marks
This work enables a pretrained text-to-video (T2V) diffusion model to be additionally conditioned on an input image (first video frame), yielding a text+image to video (TI2V) model. Other than using the pretrained T2V model, our method requires no ("zero") training or fine-tuning. The paper uses a "repeat-and-slide" method and diffusion resampling to synthesize videos from a given starting image and text describing the video content.
Paper: https://www.merl.com/publications/TR2024-059
Project page: https://merl.com/research/highlights/TI2V-Zero
2. "Long-Tailed Anomaly Detection with Learnable Class Names" by C.-H. Ho, K.-C. Peng, and N. Vasconcelos
This work aims to identify defects across various classes without relying on hard-coded class names. We introduce the concept of long-tailed anomaly detection, addressing challenges like class imbalance and dataset variability. Our proposed method combines reconstruction and semantic modules, learning pseudo-class names and utilizing a variational autoencoder for feature synthesis to improve performance in long-tailed datasets, outperforming existing methods in experiments.
Paper: https://www.merl.com/publications/TR2024-040
3. "Gear-NeRF: Free-Viewpoint Rendering and Tracking with Motion-aware Spatio-Temporal Sampling" by X. Liu, Y-W. Tai, C-T. Tang, P. Miraldo, S. Lohit, and M. Chatterjee
This work presents a new strategy for rendering dynamic scenes from novel viewpoints. Our approach is based on stratifying the scene into regions based on the extent of motion of the region, which is automatically determined. Regions with higher motion are permitted a denser spatio-temporal sampling strategy for more faithful rendering of the scene. Additionally, to the best of our knowledge, ours is the first work to enable tracking of objects in the scene from novel views - based on the preferences of a user, provided by a click.
Paper: https://www.merl.com/publications/TR2024-042
4. "SIRA: Scalable Inter-frame Relation and Association for Radar Perception" by R. Yataka, P. Wang, P. T. Boufounos, and R. Takahashi
Overcoming the limitations on radar feature extraction such as low spatial resolution, multipath reflection, and motion blurs, this paper proposes SIRA (Scalable Inter-frame Relation and Association) for scalable radar perception with two designs: 1) extended temporal relation, generalizing the existing temporal relation layer from two frames to multiple inter-frames with temporally regrouped window attention for scalability; and 2) motion consistency track with a pseudo-tracklet generated from observational data for better object association.
Paper: https://www.merl.com/publications/TR2024-041
5. "RILA: Reflective and Imaginative Language Agent for Zero-Shot Semantic Audio-Visual Navigation" by Z. Yang, J. Liu, P. Chen, A. Cherian, T. K. Marks, J. L. Roux, and C. Gan
We leverage Large Language Models (LLM) for zero-shot semantic audio visual navigation. Specifically, by employing multi-modal models to process sensory data, we instruct an LLM-based planner to actively explore the environment by adaptively evaluating and dismissing inaccurate perceptual descriptions.
Paper: https://www.merl.com/publications/TR2024-043
CVPR Workshop Papers:
1. "CoLa-SDF: Controllable Latent StyleSDF for Disentangled 3D Face Generation" by R. Dey, B. Egger, V. Boddeti, Y. Wang, and T. K. Marks
This paper proposes a new method for generating 3D faces and rendering them to images by combining the controllability of nonlinear 3DMMs with the high fidelity of implicit 3D GANs. Inspired by StyleSDF, our model uses a similar architecture but enforces the latent space to match the interpretable and physical parameters of the nonlinear 3D morphable model MOST-GAN.
Paper: https://www.merl.com/publications/TR2024-045
2. “Tracklet-based Explainable Video Anomaly Localization” by A. Singh, M. J. Jones, and E. Learned-Miller
This paper describes a new method for localizing anomalous activity in video of a scene given sample videos of normal activity from the same scene. The method is based on detecting and tracking objects in the scene and estimating high-level attributes of the objects such as their location, size, short-term trajectory and object class. These high-level attributes can then be used to detect unusual activity as well as to provide a human-understandable explanation for what is unusual about the activity.
Paper: https://www.merl.com/publications/TR2024-057
MERL co-organized workshops:
1. "Multimodal Algorithmic Reasoning Workshop" by A. Cherian, K-C. Peng, S. Lohit, M. Chatterjee, H. Zhou, K. Smith, T. K. Marks, J. Mathissen, and J. Tenenbaum
Workshop link: https://marworkshop.github.io/cvpr24/index.html
2. "The 5th Workshop on Fair, Data-Efficient, and Trusted Computer Vision" by K-C. Peng, et al.
Workshop link: https://fadetrcv.github.io/2024/
3. "SuperLoRA: Parameter-Efficient Unified Adaptation for Large Vision Models" by X. Chen, J. Liu, Y. Wang, P. Wang, M. Brand, G. Wang, and T. Koike-Akino
This paper proposes a generalized framework called SuperLoRA that unifies and extends different variants of low-rank adaptation (LoRA). Introducing new options with grouping, folding, shuffling, projection, and tensor decomposition, SuperLoRA offers high flexibility and demonstrates superior performance up to 10-fold gain in parameter efficiency for transfer learning tasks.
Paper: https://www.merl.com/publications/TR2024-062
- MERL researchers are presenting 5 conference papers, 3 workshop papers, and are co-organizing two workshops at the CVPR 2024 conference, which will be held in Seattle, June 17-21. CVPR is one of the most prestigious and competitive international conferences in computer vision. Details of MERL contributions are provided below.
See All News & Events for Moitreya -
-
Research Highlights
-
MERL Publications
- "UWAV: Uncertainty-weighted Weakly-supervised Audio-Visual Video Parsing", IEEE Conference on Computer Vision and Pattern Recognition (CVPR), June 2025.BibTeX TR2025-072 PDF
- @inproceedings{Lai2025jun,
- author = {Lai, Yung-Hsuan and Ebbers, Janek and Wang, Yu-Chiang Frank and Germain, François G and Jones, Michael J. and Chatterjee, Moitreya},
- title = {{UWAV: Uncertainty-weighted Weakly-supervised Audio-Visual Video Parsing}},
- booktitle = {IEEE Conference on Computer Vision and Pattern Recognition (CVPR)},
- year = 2025,
- month = jun,
- url = {https://www.merl.com/publications/TR2025-072}
- }
, - "FreBIS: Frequency-Based Stratification for Neural Implicit Surface Representations", IEEE Conference on Computer Vision and Pattern Recognition Workshop (CVPR), June 2025.BibTeX TR2025-074 PDF
- @inproceedings{Sawada2025jun,
- author = {Sawada, Naoko and Miraldo, Pedro and Lohit, Suhas and Marks, Tim K. and Chatterjee, Moitreya},
- title = {{FreBIS: Frequency-Based Stratification for Neural Implicit Surface Representations}},
- booktitle = {IEEE Conference on Computer Vision and Pattern Recognition Workshop (CVPR)},
- year = 2025,
- month = jun,
- url = {https://www.merl.com/publications/TR2025-074}
- }
, - "Improving Open-World Object Localization by Discovering Background", CVPR Workshop on Domain Generalization: Evolution, Breakthroughs and Future Horizon, May 2025.BibTeX TR2025-058 PDF
- @inproceedings{Singh2025may,
- author = {Singh, Ashish and Jones, Michael J. and Peng, Kuan-Chuan and Chatterjee, Moitreya and Cherian, Anoop and Learned-Miller, Erik},
- title = {{Improving Open-World Object Localization by Discovering Background}},
- booktitle = {CVPR Workshop on Domain Generalization: Evolution, Breakthroughs and Future Horizon},
- year = 2025,
- month = may,
- url = {https://www.merl.com/publications/TR2025-058}
- }
, - "Programmatic Video Prediction Using Large Language Models", International Conference on Learning Representations Workshops (ICLRW), April 2025.BibTeX TR2025-049 PDF
- @inproceedings{Tang2025apr,
- author = {Tang, Hao and Ellis, Kevin and Lohit, Suhas and Jones, Michael J. and Chatterjee, Moitreya},
- title = {{Programmatic Video Prediction Using Large Language Models}},
- booktitle = {International Conference on Learning Representations Workshops (ICLRW)},
- year = 2025,
- month = apr,
- url = {https://www.merl.com/publications/TR2025-049}
- }
, - "A Probability-guided Sampler for Neural Implicit Surface Rendering", European Conference on Computer Vision (ECCV), Leonardis, A. and Ricci, E. and Roth, S. and Russakovsky, O., Sattler, T. and Varol, G., Eds., DOI: 10.1007/978-3-031-72913-3_10, September 2024, pp. 164-182.BibTeX TR2024-129 PDF Video
- @inproceedings{Pais2024sep,
- author = {Pais, Goncalo and Piedade, Valter and Chatterjee, Moitreya and Greiff, Marcus and Miraldo, Pedro},
- title = {{A Probability-guided Sampler for Neural Implicit Surface Rendering}},
- booktitle = {European Conference on Computer Vision (ECCV)},
- year = 2024,
- editor = {Leonardis, A. and Ricci, E. and Roth, S. and Russakovsky, O., Sattler, T. and Varol, G.},
- pages = {164--182},
- month = sep,
- publisher = {Springer, Cham},
- doi = {10.1007/978-3-031-72913-3_10},
- isbn = {978-3-031-72913-3},
- url = {https://www.merl.com/publications/TR2024-129}
- }
,
- "UWAV: Uncertainty-weighted Weakly-supervised Audio-Visual Video Parsing", IEEE Conference on Computer Vision and Pattern Recognition (CVPR), June 2025.
-
Other Publications
- "A hierarchical variational neural uncertainty model for stochastic video prediction", Proceedings of the IEEE/CVF International Conference on Computer Vision, 2021, pp. 9751-9761.BibTeX
- @Inproceedings{chatterjee2021hierarchical,
- author = {Chatterjee, Moitreya and Ahuja, Narendra and Cherian, Anoop},
- title = {A hierarchical variational neural uncertainty model for stochastic video prediction},
- booktitle = {Proceedings of the IEEE/CVF International Conference on Computer Vision},
- year = 2021,
- pages = {9751--9761}
- }
, - "Visual scene graphs for audio source separation", Proceedings of the IEEE/CVF International Conference on Computer Vision, 2021, pp. 1204-1213.BibTeX
- @Inproceedings{chatterjee2021visual,
- author = {Chatterjee, Moitreya and Le Roux, Jonathan and Ahuja, Narendra and Cherian, Anoop},
- title = {Visual scene graphs for audio source separation},
- booktitle = {Proceedings of the IEEE/CVF International Conference on Computer Vision},
- year = 2021,
- pages = {1204--1213}
- }
, - "Dynamic graph representation learning for video dialog via multi-modal shuffled transformers", Proceedings of the AAAI Conference on Artificial Intelligence, 2021, vol. 35, pp. 1415-1423.BibTeX
- @Inproceedings{geng2021dynamic,
- author = {Geng, Shijie and Gao, Peng and Chatterjee, Moitreya and Hori, Chiori and Le Roux, Jonathan and Zhang, Yongfeng and Li, Hongsheng and Cherian, Anoop},
- title = {Dynamic graph representation learning for video dialog via multi-modal shuffled transformers},
- booktitle = {Proceedings of the AAAI Conference on Artificial Intelligence},
- year = 2021,
- volume = 35,
- number = 2,
- pages = {1415--1423}
- }
, - "Sound2sight: Generating visual dynamics from sound and context", European Conference on Computer Vision, 2020, pp. 701-719.BibTeX
- @Inproceedings{chatterjee2020sound2sight,
- author = {Chatterjee, Moitreya and Cherian, Anoop},
- title = {Sound2sight: Generating visual dynamics from sound and context},
- booktitle = {European Conference on Computer Vision},
- year = 2020,
- pages = {701--719},
- organization = {Springer}
- }
, - "Coreset-based neural network compression", Proceedings of the European Conference on Computer Vision (ECCV), 2018, pp. 454-470.BibTeX
- @Inproceedings{dubey2018coreset,
- author = {Dubey, Abhimanyu and Chatterjee, Moitreya and Ahuja, Narendra},
- title = {Coreset-based neural network compression},
- booktitle = {Proceedings of the European Conference on Computer Vision (ECCV)},
- year = 2018,
- pages = {454--470}
- }
, - "Deep neural networks with inexact matching for person re-identification", Advances in neural information processing systems, Vol. 29, 2016.BibTeX
- @Article{subramaniam2016deep,
- author = {Subramaniam, Arulkumar and Chatterjee, Moitreya and Mittal, Anurag},
- title = {Deep neural networks with inexact matching for person re-identification},
- journal = {Advances in neural information processing systems},
- year = 2016,
- volume = 29
- }
, - "Combining two perspectives on classifying multimodal data for recognizing speaker traits", Proceedings of the 2015 ACM on International Conference on Multimodal Interaction, 2015, pp. 7-14.BibTeX
- @Inproceedings{chatterjee2015combining,
- author = {Chatterjee, Moitreya and Park, Sunghyun and Morency, Louis-Philippe and Scherer, Stefan},
- title = {Combining two perspectives on classifying multimodal data for recognizing speaker traits},
- booktitle = {Proceedings of the 2015 ACM on International Conference on Multimodal Interaction},
- year = 2015,
- pages = {7--14}
- }
,
- "A hierarchical variational neural uncertainty model for stochastic video prediction", Proceedings of the IEEE/CVF International Conference on Computer Vision, 2021, pp. 9751-9761.
-
Software & Data Downloads
-
Videos
-
MERL Issued Patents
-
Title: "A Method and System for Scene-Aware Audio-Video Representation"
Inventors: Cherian, Anoop; Chatterjee, Moitreya; Le Roux, Jonathan
Patent No.: 12,056,213
Issue Date: Aug 6, 2024
-
Title: "A Method and System for Scene-Aware Audio-Video Representation"