Ye Wang
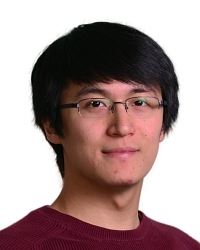
- Phone: 617-621-7521
- Email:
-
Position:
Research / Technical Staff
Senior Principal Research Scientist -
Education:
Ph.D., Boston University, 2011 -
Research Areas:
External Links:
Ye's Quick Links
-
Biography
Ye was a member of the Information Systems and Sciences Laboratory at Boston University, where he studied information-theoretically secure multiparty computation. His current research interests include information security, biometric authentication, and data privacy.
-
Recent News & Events
-
NEWS MERL Researchers to Present 2 Conference and 11 Workshop Papers at NeurIPS 2024 Date: December 10, 2024 - December 15, 2024
Where: Advances in Neural Processing Systems (NeurIPS)
MERL Contacts: Petros T. Boufounos; Matthew Brand; Ankush Chakrabarty; Anoop Cherian; François Germain; Toshiaki Koike-Akino; Christopher R. Laughman; Jonathan Le Roux; Jing Liu; Suhas Lohit; Tim K. Marks; Yoshiki Masuyama; Kieran Parsons; Kuan-Chuan Peng; Diego Romeres; Pu (Perry) Wang; Ye Wang; Gordon Wichern
Research Areas: Artificial Intelligence, Communications, Computational Sensing, Computer Vision, Control, Data Analytics, Dynamical Systems, Machine Learning, Multi-Physical Modeling, Optimization, Robotics, Signal Processing, Speech & Audio, Human-Computer Interaction, Information SecurityBrief- MERL researchers will attend and present the following papers at the 2024 Advances in Neural Processing Systems (NeurIPS) Conference and Workshops.
1. "RETR: Multi-View Radar Detection Transformer for Indoor Perception" by Ryoma Yataka (Mitsubishi Electric), Adriano Cardace (Bologna University), Perry Wang (Mitsubishi Electric Research Laboratories), Petros Boufounos (Mitsubishi Electric Research Laboratories), Ryuhei Takahashi (Mitsubishi Electric). Main Conference. https://neurips.cc/virtual/2024/poster/95530
2. "Evaluating Large Vision-and-Language Models on Children's Mathematical Olympiads" by Anoop Cherian (Mitsubishi Electric Research Laboratories), Kuan-Chuan Peng (Mitsubishi Electric Research Laboratories), Suhas Lohit (Mitsubishi Electric Research Laboratories), Joanna Matthiesen (Math Kangaroo USA), Kevin Smith (Massachusetts Institute of Technology), Josh Tenenbaum (Massachusetts Institute of Technology). Main Conference, Datasets and Benchmarks track. https://neurips.cc/virtual/2024/poster/97639
3. "Probabilistic Forecasting for Building Energy Systems: Are Time-Series Foundation Models The Answer?" by Young-Jin Park (Massachusetts Institute of Technology), Jing Liu (Mitsubishi Electric Research Laboratories), François G Germain (Mitsubishi Electric Research Laboratories), Ye Wang (Mitsubishi Electric Research Laboratories), Toshiaki Koike-Akino (Mitsubishi Electric Research Laboratories), Gordon Wichern (Mitsubishi Electric Research Laboratories), Navid Azizan (Massachusetts Institute of Technology), Christopher R. Laughman (Mitsubishi Electric Research Laboratories), Ankush Chakrabarty (Mitsubishi Electric Research Laboratories). Time Series in the Age of Large Models Workshop.
4. "Forget to Flourish: Leveraging Model-Unlearning on Pretrained Language Models for Privacy Leakage" by Md Rafi Ur Rashid (Penn State University), Jing Liu (Mitsubishi Electric Research Laboratories), Toshiaki Koike-Akino (Mitsubishi Electric Research Laboratories), Shagufta Mehnaz (Penn State University), Ye Wang (Mitsubishi Electric Research Laboratories). Workshop on Red Teaming GenAI: What Can We Learn from Adversaries?
5. "Spatially-Aware Losses for Enhanced Neural Acoustic Fields" by Christopher Ick (New York University), Gordon Wichern (Mitsubishi Electric Research Laboratories), Yoshiki Masuyama (Mitsubishi Electric Research Laboratories), François G Germain (Mitsubishi Electric Research Laboratories), Jonathan Le Roux (Mitsubishi Electric Research Laboratories). Audio Imagination Workshop.
6. "FV-NeRV: Neural Compression for Free Viewpoint Videos" by Sorachi Kato (Osaka University), Takuya Fujihashi (Osaka University), Toshiaki Koike-Akino (Mitsubishi Electric Research Laboratories), Takashi Watanabe (Osaka University). Machine Learning and Compression Workshop.
7. "GPT Sonography: Hand Gesture Decoding from Forearm Ultrasound Images via VLM" by Keshav Bimbraw (Worcester Polytechnic Institute), Ye Wang (Mitsubishi Electric Research Laboratories), Jing Liu (Mitsubishi Electric Research Laboratories), Toshiaki Koike-Akino (Mitsubishi Electric Research Laboratories). AIM-FM: Advancements In Medical Foundation Models: Explainability, Robustness, Security, and Beyond Workshop.
8. "Smoothed Embeddings for Robust Language Models" by Hase Ryo (Mitsubishi Electric), Md Rafi Ur Rashid (Penn State University), Ashley Lewis (Ohio State University), Jing Liu (Mitsubishi Electric Research Laboratories), Toshiaki Koike-Akino (Mitsubishi Electric Research Laboratories), Kieran Parsons (Mitsubishi Electric Research Laboratories), Ye Wang (Mitsubishi Electric Research Laboratories). Safe Generative AI Workshop.
9. "Slaying the HyDRA: Parameter-Efficient Hyper Networks with Low-Displacement Rank Adaptation" by Xiangyu Chen (University of Kansas), Ye Wang (Mitsubishi Electric Research Laboratories), Matthew Brand (Mitsubishi Electric Research Laboratories), Pu Wang (Mitsubishi Electric Research Laboratories), Jing Liu (Mitsubishi Electric Research Laboratories), Toshiaki Koike-Akino (Mitsubishi Electric Research Laboratories). Workshop on Adaptive Foundation Models.
10. "Preference-based Multi-Objective Bayesian Optimization with Gradients" by Joshua Hang Sai Ip (University of California Berkeley), Ankush Chakrabarty (Mitsubishi Electric Research Laboratories), Ali Mesbah (University of California Berkeley), Diego Romeres (Mitsubishi Electric Research Laboratories). Workshop on Bayesian Decision-Making and Uncertainty. Lightning talk spotlight.
11. "TR-BEACON: Shedding Light on Efficient Behavior Discovery in High-Dimensions with Trust-Region-based Bayesian Novelty Search" by Wei-Ting Tang (Ohio State University), Ankush Chakrabarty (Mitsubishi Electric Research Laboratories), Joel A. Paulson (Ohio State University). Workshop on Bayesian Decision-Making and Uncertainty.
12. "MEL-PETs Joint-Context Attack for the NeurIPS 2024 LLM Privacy Challenge Red Team Track" by Ye Wang (Mitsubishi Electric Research Laboratories), Tsunato Nakai (Mitsubishi Electric), Jing Liu (Mitsubishi Electric Research Laboratories), Toshiaki Koike-Akino (Mitsubishi Electric Research Laboratories), Kento Oonishi (Mitsubishi Electric), Takuya Higashi (Mitsubishi Electric). LLM Privacy Challenge. Special Award for Practical Attack.
13. "MEL-PETs Defense for the NeurIPS 2024 LLM Privacy Challenge Blue Team Track" by Jing Liu (Mitsubishi Electric Research Laboratories), Ye Wang (Mitsubishi Electric Research Laboratories), Toshiaki Koike-Akino (Mitsubishi Electric Research Laboratories), Tsunato Nakai (Mitsubishi Electric), Kento Oonishi (Mitsubishi Electric), Takuya Higashi (Mitsubishi Electric). LLM Privacy Challenge. Won 3rd Place Award.
MERL members also contributed to the organization of the Multimodal Algorithmic Reasoning (MAR) Workshop (https://marworkshop.github.io/neurips24/). Organizers: Anoop Cherian (Mitsubishi Electric Research Laboratories), Kuan-Chuan Peng (Mitsubishi Electric Research Laboratories), Suhas Lohit (Mitsubishi Electric Research Laboratories), Honglu Zhou (Salesforce Research), Kevin Smith (Massachusetts Institute of Technology), Tim K. Marks (Mitsubishi Electric Research Laboratories), Juan Carlos Niebles (Salesforce AI Research), Petar Veličković (Google DeepMind).
- MERL researchers will attend and present the following papers at the 2024 Advances in Neural Processing Systems (NeurIPS) Conference and Workshops.
-
NEWS MERL Papers and Workshops at CVPR 2024 Date: June 17, 2024 - June 21, 2024
Where: Seattle, WA
MERL Contacts: Petros T. Boufounos; Moitreya Chatterjee; Anoop Cherian; Michael J. Jones; Toshiaki Koike-Akino; Jonathan Le Roux; Suhas Lohit; Tim K. Marks; Pedro Miraldo; Jing Liu; Kuan-Chuan Peng; Pu (Perry) Wang; Ye Wang; Matthew Brand
Research Areas: Artificial Intelligence, Computational Sensing, Computer Vision, Machine Learning, Speech & AudioBrief- MERL researchers are presenting 5 conference papers, 3 workshop papers, and are co-organizing two workshops at the CVPR 2024 conference, which will be held in Seattle, June 17-21. CVPR is one of the most prestigious and competitive international conferences in computer vision. Details of MERL contributions are provided below.
CVPR Conference Papers:
1. "TI2V-Zero: Zero-Shot Image Conditioning for Text-to-Video Diffusion Models" by H. Ni, B. Egger, S. Lohit, A. Cherian, Y. Wang, T. Koike-Akino, S. X. Huang, and T. K. Marks
This work enables a pretrained text-to-video (T2V) diffusion model to be additionally conditioned on an input image (first video frame), yielding a text+image to video (TI2V) model. Other than using the pretrained T2V model, our method requires no ("zero") training or fine-tuning. The paper uses a "repeat-and-slide" method and diffusion resampling to synthesize videos from a given starting image and text describing the video content.
Paper: https://www.merl.com/publications/TR2024-059
Project page: https://merl.com/research/highlights/TI2V-Zero
2. "Long-Tailed Anomaly Detection with Learnable Class Names" by C.-H. Ho, K.-C. Peng, and N. Vasconcelos
This work aims to identify defects across various classes without relying on hard-coded class names. We introduce the concept of long-tailed anomaly detection, addressing challenges like class imbalance and dataset variability. Our proposed method combines reconstruction and semantic modules, learning pseudo-class names and utilizing a variational autoencoder for feature synthesis to improve performance in long-tailed datasets, outperforming existing methods in experiments.
Paper: https://www.merl.com/publications/TR2024-040
3. "Gear-NeRF: Free-Viewpoint Rendering and Tracking with Motion-aware Spatio-Temporal Sampling" by X. Liu, Y-W. Tai, C-T. Tang, P. Miraldo, S. Lohit, and M. Chatterjee
This work presents a new strategy for rendering dynamic scenes from novel viewpoints. Our approach is based on stratifying the scene into regions based on the extent of motion of the region, which is automatically determined. Regions with higher motion are permitted a denser spatio-temporal sampling strategy for more faithful rendering of the scene. Additionally, to the best of our knowledge, ours is the first work to enable tracking of objects in the scene from novel views - based on the preferences of a user, provided by a click.
Paper: https://www.merl.com/publications/TR2024-042
4. "SIRA: Scalable Inter-frame Relation and Association for Radar Perception" by R. Yataka, P. Wang, P. T. Boufounos, and R. Takahashi
Overcoming the limitations on radar feature extraction such as low spatial resolution, multipath reflection, and motion blurs, this paper proposes SIRA (Scalable Inter-frame Relation and Association) for scalable radar perception with two designs: 1) extended temporal relation, generalizing the existing temporal relation layer from two frames to multiple inter-frames with temporally regrouped window attention for scalability; and 2) motion consistency track with a pseudo-tracklet generated from observational data for better object association.
Paper: https://www.merl.com/publications/TR2024-041
5. "RILA: Reflective and Imaginative Language Agent for Zero-Shot Semantic Audio-Visual Navigation" by Z. Yang, J. Liu, P. Chen, A. Cherian, T. K. Marks, J. L. Roux, and C. Gan
We leverage Large Language Models (LLM) for zero-shot semantic audio visual navigation. Specifically, by employing multi-modal models to process sensory data, we instruct an LLM-based planner to actively explore the environment by adaptively evaluating and dismissing inaccurate perceptual descriptions.
Paper: https://www.merl.com/publications/TR2024-043
CVPR Workshop Papers:
1. "CoLa-SDF: Controllable Latent StyleSDF for Disentangled 3D Face Generation" by R. Dey, B. Egger, V. Boddeti, Y. Wang, and T. K. Marks
This paper proposes a new method for generating 3D faces and rendering them to images by combining the controllability of nonlinear 3DMMs with the high fidelity of implicit 3D GANs. Inspired by StyleSDF, our model uses a similar architecture but enforces the latent space to match the interpretable and physical parameters of the nonlinear 3D morphable model MOST-GAN.
Paper: https://www.merl.com/publications/TR2024-045
2. “Tracklet-based Explainable Video Anomaly Localization” by A. Singh, M. J. Jones, and E. Learned-Miller
This paper describes a new method for localizing anomalous activity in video of a scene given sample videos of normal activity from the same scene. The method is based on detecting and tracking objects in the scene and estimating high-level attributes of the objects such as their location, size, short-term trajectory and object class. These high-level attributes can then be used to detect unusual activity as well as to provide a human-understandable explanation for what is unusual about the activity.
Paper: https://www.merl.com/publications/TR2024-057
MERL co-organized workshops:
1. "Multimodal Algorithmic Reasoning Workshop" by A. Cherian, K-C. Peng, S. Lohit, M. Chatterjee, H. Zhou, K. Smith, T. K. Marks, J. Mathissen, and J. Tenenbaum
Workshop link: https://marworkshop.github.io/cvpr24/index.html
2. "The 5th Workshop on Fair, Data-Efficient, and Trusted Computer Vision" by K-C. Peng, et al.
Workshop link: https://fadetrcv.github.io/2024/
3. "SuperLoRA: Parameter-Efficient Unified Adaptation for Large Vision Models" by X. Chen, J. Liu, Y. Wang, P. Wang, M. Brand, G. Wang, and T. Koike-Akino
This paper proposes a generalized framework called SuperLoRA that unifies and extends different variants of low-rank adaptation (LoRA). Introducing new options with grouping, folding, shuffling, projection, and tensor decomposition, SuperLoRA offers high flexibility and demonstrates superior performance up to 10-fold gain in parameter efficiency for transfer learning tasks.
Paper: https://www.merl.com/publications/TR2024-062
- MERL researchers are presenting 5 conference papers, 3 workshop papers, and are co-organizing two workshops at the CVPR 2024 conference, which will be held in Seattle, June 17-21. CVPR is one of the most prestigious and competitive international conferences in computer vision. Details of MERL contributions are provided below.
See All News & Events for Ye -
-
Awards
-
AWARD MERL Wins Awards at NeurIPS LLM Privacy Challenge Date: December 15, 2024
Awarded to: Jing Liu, Ye Wang, Toshiaki Koike-Akino, Tsunato Nakai, Kento Oonishi, Takuya Higashi
MERL Contacts: Toshiaki Koike-Akino; Jing Liu; Ye Wang
Research Areas: Artificial Intelligence, Machine Learning, Information SecurityBrief- The Mitsubishi Electric Privacy Enhancing Technologies (MEL-PETs) team, consisting of a collaboration of MERL and Mitsubishi Electric researchers, won awards at the NeurIPS 2024 Large Language Model (LLM) Privacy Challenge. In the Blue Team track of the challenge, we won the 3rd Place Award, and in the Red Team track, we won the Special Award for Practical Attack.
-
AWARD MERL’s Paper on Wi-Fi Sensing Earns Top 3% Paper Recognition at ICASSP 2023, Selected as a Best Student Paper Award Finalist Date: June 9, 2023
Awarded to: Cristian J. Vaca-Rubio, Pu Wang, Toshiaki Koike-Akino, Ye Wang, Petros Boufounos and Petar Popovski
MERL Contacts: Petros T. Boufounos; Toshiaki Koike-Akino; Pu (Perry) Wang; Ye Wang
Research Areas: Artificial Intelligence, Communications, Computational Sensing, Dynamical Systems, Machine Learning, Signal ProcessingBrief- A MERL Paper on Wi-Fi sensing was recognized as a Top 3% Paper among all 2709 accepted papers at the 2023 IEEE International Conference on Acoustics, Speech and Signal Processing (ICASSP 2023). Co-authored by Cristian Vaca-Rubio and Petar Popovski from Aalborg University, Denmark, and MERL researchers Pu Wang, Toshiaki Koike-Akino, Ye Wang, and Petros Boufounos, the paper "MmWave Wi-Fi Trajectory Estimation with Continous-Time Neural Dynamic Learning" was also a Best Student Paper Award finalist.
Performed during Cristian’s stay at MERL first as a visiting Marie Skłodowska-Curie Fellow and then as a full-time intern in 2022, this work capitalizes on standards-compliant Wi-Fi signals to perform indoor localization and sensing. The paper uses a neural dynamic learning framework to address technical issues such as low sampling rate and irregular sampling intervals.
ICASSP, a flagship conference of the IEEE Signal Processing Society (SPS), was hosted on the Greek island of Rhodes from June 04 to June 10, 2023. ICASSP 2023 marked the largest ICASSP in history, boasting over 4000 participants and 6128 submitted papers, out of which 2709 were accepted.
- A MERL Paper on Wi-Fi sensing was recognized as a Top 3% Paper among all 2709 accepted papers at the 2023 IEEE International Conference on Acoustics, Speech and Signal Processing (ICASSP 2023). Co-authored by Cristian Vaca-Rubio and Petar Popovski from Aalborg University, Denmark, and MERL researchers Pu Wang, Toshiaki Koike-Akino, Ye Wang, and Petros Boufounos, the paper "MmWave Wi-Fi Trajectory Estimation with Continous-Time Neural Dynamic Learning" was also a Best Student Paper Award finalist.
-
AWARD MERL Ranked 1st Place in Cross-Subject Transfer Learning Task and 4th Place Overall at the NeurIPS2021 BEETL Competition for EEG Transfer Learning. Date: November 11, 2021
Awarded to: Niklas Smedemark-Margulies, Toshiaki Koike-Akino, Ye Wang, Deniz Erdogmus
MERL Contacts: Toshiaki Koike-Akino; Ye Wang
Research Areas: Artificial Intelligence, Signal Processing, Human-Computer InteractionBrief- The MERL Signal Processing group achieved first place in the cross-subject transfer learning task and fourth place overall in the NeurIPS 2021 BEETL AI Challenge for EEG Transfer Learning. The team included Niklas Smedemark-Margulies (intern from Northeastern University), Toshiaki Koike-Akino, Ye Wang, and Prof. Deniz Erdogmus (Northeastern University). The challenge addresses two types of transfer learning tasks for EEG Biosignals: a homogeneous transfer learning task for cross-subject domain adaptation; and a heterogeneous transfer learning task for cross-data domain adaptation. There were 110+ registered teams in this competition, MERL ranked 1st in the homogeneous transfer learning task, 7th place in the heterogeneous transfer learning task, and 4th place for the combined overall score. For the homogeneous transfer learning task, MERL developed a new pre-shot learning framework based on feature disentanglement techniques for robustness against inter-subject variation to enable calibration-free brain-computer interfaces (BCI). MERL is invited to present our pre-shot learning technique at the NeurIPS 2021 workshop.
-
-
Research Highlights
-
Internships with Ye
-
CI0139: Internship - Trustworthy and General AI
MERL is seeking passionate and skilled research interns to join our team focused on developing trustworthy, safe, and robust machine learning technologies towards realizing more capable, general agents. This is an exciting opportunity to make an impact on the field of AI safety and generalization, with the aim of publishing at leading AI research venues.
What We're Looking For:
- Advanced research experience with generative models related to the topics of AI safety, robustness, trustworthiness, and/or more capable agents.
- Hands-on skills for large language models (LLM), vision language models (VLM), large multi-modal models (LMM), foundation models (FoMo)
- Deep understanding of state-of-the-art machine learning methods
- Proficiency in Python and PyTorch
- Familiarity with other relevant deep learning frameworks
- Ph.D. candidates who have completed at least half of their program
Internship Details:
- Duration: approximately 3 months
- Flexible start dates available
- Objective: publish research results at leading AI research venues
If you're a highly motivated individual with a passion for tackling AI safety and privacy challenges, we want to hear from you! This internship offers a unique chance to work on meaningful AI research projects, combined with the opportunity to publish and add to your thesis.
-
-
MERL Publications
- "Slaying the HyDRA: Parameter-Efficient Hyper Networks with Low-Displacement Rank Adaptation", Advances in Neural Information Processing Systems (NeurIPS), December 2024.BibTeX TR2024-157 PDF Presentation
- @inproceedings{Chen2024dec,
- author = {{Chen, Xiangyu and Wang, Ye and Brand, Matthew and Wang, Pu and Liu, Jing and Koike-Akino, Toshiaki}},
- title = {Slaying the HyDRA: Parameter-Efficient Hyper Networks with Low-Displacement Rank Adaptation},
- booktitle = {Workshop on Adaptive Foundation Models: Evolving AI for Personalized and Efficient Learning at Neural Information Processing Systems (NeurIPS)},
- year = 2024,
- month = dec,
- url = {https://www.merl.com/publications/TR2024-157}
- }
, - "GPT Sonograpy: Hand Gesture Decoding from Forearm Ultrasound Images via VLM", Advances in Neural Information Processing Systems (NeurIPS), December 2024.BibTeX TR2024-175 PDF Presentation
- @inproceedings{Bimbraw2024dec,
- author = {{Bimbraw, Keshav and Wang, Ye and Liu, Jing and Koike-Akino, Toshiaki}},
- title = {GPT Sonograpy: Hand Gesture Decoding from Forearm Ultrasound Images via VLM},
- booktitle = {Advancements In Medical Foundation Models: Explainability, Robustness, Security, and Beyond Workshop at Neural Information Processing Systems (NeurIPS)},
- year = 2024,
- month = dec,
- url = {https://www.merl.com/publications/TR2024-175}
- }
, - "Probabilistic Forecasting for Building Energy Systems: Are Time-Series Foundation Models The Answer?", Advances in Neural Information Processing Systems (NeurIPS), December 2024.BibTeX TR2025-001 PDF
- @inproceedings{Park2024dec,
- author = {Park, Young-Jin and Germain, François G and Liu, Jing and Wang, Ye and Koike-Akino, Toshiaki and Wichern, Gordon and Christopher R. and Azizan, Navid and Laughman, Chakrabarty, Ankush}},
- title = {Probabilistic Forecasting for Building Energy Systems: Are Time-Series Foundation Models The Answer?},
- booktitle = {Advances in Neural Information Processing Systems (NeurIPS)},
- year = 2024,
- month = dec,
- url = {https://www.merl.com/publications/TR2025-001}
- }
, - "MEL-PETs Defense for the NeurIPS 2024 LLM Privacy Challenge Blue Team Track", LLM Privacy Challenge at Neural Information Processing Systems (NeurIPS) 2024, December 2024.BibTeX TR2024-166 PDF Video Presentation
- @inproceedings{Liu2024dec,
- author = {{Liu, Jing and Wang, Ye and Koike-Akino, Toshiaki and Nakai, Tsunato and Oonishi, Kento and Higashi, Takuya}},
- title = {MEL-PETs Defense for the NeurIPS 2024 LLM Privacy Challenge Blue Team Track},
- booktitle = {LLM Privacy Challenge at Neural Information Processing Systems (NeurIPS) 2024},
- year = 2024,
- month = dec,
- url = {https://www.merl.com/publications/TR2024-166}
- }
, - "MEL-PETs Joint-Context Attack for the NeurIPS 2024 LLM Privacy Challenge Red Team Track", LLM Privacy Challenge at Neural Information Processing Systems (NeurIPS), December 2024.BibTeX TR2024-165 PDF Video Presentation
- @inproceedings{Wang2024dec2,
- author = {{Wang, Ye and Nakai, Tsunato and Liu, Jing and Koike-Akino, Toshiaki and Oonishi, Kento and Higashi, Takuya}},
- title = {MEL-PETs Joint-Context Attack for the NeurIPS 2024 LLM Privacy Challenge Red Team Track},
- booktitle = {LLM Privacy Challenge at Neural Information Processing Systems (NeurIPS)},
- year = 2024,
- month = dec,
- url = {https://www.merl.com/publications/TR2024-165}
- }
,
- "Slaying the HyDRA: Parameter-Efficient Hyper Networks with Low-Displacement Rank Adaptation", Advances in Neural Information Processing Systems (NeurIPS), December 2024.
-
Software & Data Downloads
-
Stabilizing Subject Transfer in EEG Classification with Divergence Estimation -
MEL-PETs Joint-Context Attack for LLM Privacy Challenge -
MEL-PETs Defense for LLM Privacy Challenge -
Steered Diffusion -
Nonparametric Score Estimators -
3D MOrphable STyleGAN -
Landmarks’ Location, Uncertainty, and Visibility Likelihood
-
-
Videos
-
MERL Issued Patents
-
Title: "Automated Construction of Neural Network Architecture with Bayesian Graph Exploration"
Inventors: Koike-Akino, Toshiaki; Wang, Ye; Demir, Andac; Erdogmus, Deniz
Patent No.: 12,061,985
Issue Date: Aug 13, 2024 -
Title: "Anomaly Detection and Diagnosis in Factory Automation System using Pre-Processed Time-Delay Neural Network with Loss Function Adaptation"
Inventors: Guo, Jianlin; Liu, Bryan; Koike-Akino, Toshiaki; Wang, Ye; Kim, Kyeong-Jin; Parsons, Kieran; Orlik, Philip V.
Patent No.: 12,007,760
Issue Date: Jun 11, 2024 -
Title: "Multi-Band Wi-Fi Fusion for WLAN Sensing"
Inventors: Wang, Pu; Yu, Jianyuan; Koike-Akino, Toshiaki; Wang, Ye; Orlik, Philip V.
Patent No.: 11,902,811
Issue Date: Feb 13, 2024 -
Title: "Apparatus and Method for Anomaly Detection"
Inventors: Wang, Ye; Kim, Kyeong-Jin; Wang, Xiao
Patent No.: 11,843,623
Issue Date: Dec 12, 2023 -
Title: "System and Method for Manipulating Two-Dimensional (2D) Images of Three-Dimensional (3D) Objects"
Inventors: Marks, Tim; Medin, Safa; Cherian, Anoop; Wang, Ye
Patent No.: 11,663,798
Issue Date: May 30, 2023 -
Title: "Non-Uniform Regularization in Artificial Neural Networks for Adaptable Scaling"
Inventors: Wang, Ye; Koike-Akino, Toshiaki
Patent No.: 11,651,225
Issue Date: May 16, 2023 -
Title: "Protograph Quasi-Cyclic Polar Codes and Related Low-Density Generator Matrix Family"
Inventors: Koike-Akino, Toshiaki; Wang, Ye
Patent No.: 11,463,114
Issue Date: Oct 4, 2022 -
Title: "Battery Diagnostic System for Estimating Remaining useful Life (RUL) of a Battery"
Inventors: Gorrachategui, Ivan Sanz; Pajovic, Milutin; Wang, Ye
Patent No.: 11,346,891
Issue Date: May 31, 2022 -
Title: "Generative Model for Inverse Design of Materials, Devices, and Structures"
Inventors: Kojima, Keisuke; Tang, Yingheng; Koike-Akino, Toshiaki; Wang, Ye
Patent No.: 11,251,896
Issue Date: Feb 15, 2022 -
Title: "DATA-DRIVEN PRIVACY-PRESERVING COMMUNICATION"
Inventors: Wang, Ye; Ishwar, Prakash; Tripathy, Ardhendu S
Patent No.: 11,132,453
Issue Date: Sep 28, 2021 -
Title: "Irregular Polar Code Encoding"
Inventors: Koike-Akino, Toshiaki; Wang, Ye; Draper, Stark C.
Patent No.: 10,862,621
Issue Date: Dec 8, 2020 -
Title: "Method and Systems using Privacy-Preserving Analytics for Aggregate Data"
Inventors: Wang, Ye; Raval, Nisarg Jagdishbhai; Ishwar, Prakash
Patent No.: 10,452,865
Issue Date: Oct 22, 2019 -
Title: "Irregular Polar Code Encoding"
Inventors: Koike-Akino, Toshiaki; Wang, Ye; Draper, Stark C.
Patent No.: 10,313,056
Issue Date: Jun 4, 2019 -
Title: "Soft-Output Decoding of Codewords Encoded with Polar Code"
Inventors: Wang, Ye; Koike-Akino, Toshiaki; Draper, Stark C.
Patent No.: 10,312,946
Issue Date: Jun 4, 2019 -
Title: "Method and Systems using Privacy-Preserving Analytics for Aggregate Data"
Inventors: Wang, Ye; Hattori, Mitsuhiro; Shimizu, Rina; Hirano, Takato; Matsuda, Nori
Patent No.: 10,216,959
Issue Date: Feb 26, 2019 -
Title: "Privacy Preserving Statistical Analysis on Distributed Databases"
Inventors: Wang, Ye; Lin, Bing-Rong; Rane, Shantanu D.
Patent No.: 10,146,958
Issue Date: Dec 4, 2018 -
Title: "Method and System for Determining Hidden States of a Machine using Privacy-Preserving Distributed Data Analytics and a Semi-trusted Server and a Third-Party"
Inventors: Wang, Ye
Patent No.: 9,471,810
Issue Date: Oct 18, 2016 -
Title: "Method for Determining Hidden States of Systems using Privacy-Preserving Distributed Data Analytics"
Inventors: Wang, Ye; Xie, Qian; Rane, Shantanu D.
Patent No.: 9,246,978
Issue Date: Jan 26, 2016 -
Title: "Privacy Preserving Statistical Analysis for Distributed Databases"
Inventors: Wang, Ye; Lin, Bing-Rong; Rane, Shantanu D.
Patent No.: 8,893,292
Issue Date: Nov 18, 2014 -
Title: "Secure Multi-Party Computation of Normalized Sum-Type Functions"
Inventors: Rane, Shantanu D.; Sun, Wei; Wang, Ye
Patent No.: 8,473,537
Issue Date: Jun 25, 2013
-
Title: "Automated Construction of Neural Network Architecture with Bayesian Graph Exploration"