Michael J. Jones
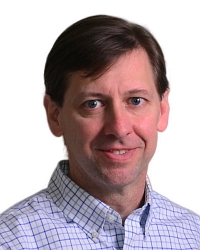
- Phone: 617-621-7587
- Email:
-
Position:
Research / Technical Staff
Senior Principal Research Scientist -
Education:
Ph.D., Massachusetts Institute of Technology, 1997 -
Research Areas:
External Links:
Mike's Quick Links
-
Biography
Mike's main areas of interest are computer vision, machine learning and data mining. He has focused on algorithms for detecting and analyzing people in images and video including face detection and recognition and pedestrian detection. He is a co-inventor of the popular Viola-Jones face detection method. Mike has been awarded the Marr Prize at ICCV and the Longuet-Higgins Prize at CVPR.
-
Recent News & Events
-
NEWS MERL Papers and Workshops at CVPR 2024 Date: June 17, 2024 - June 21, 2024
Where: Seattle, WA
MERL Contacts: Petros T. Boufounos; Moitreya Chatterjee; Anoop Cherian; Michael J. Jones; Toshiaki Koike-Akino; Jonathan Le Roux; Suhas Lohit; Tim K. Marks; Pedro Miraldo; Jing Liu; Kuan-Chuan Peng; Pu (Perry) Wang; Ye Wang; Matthew Brand
Research Areas: Artificial Intelligence, Computational Sensing, Computer Vision, Machine Learning, Speech & AudioBrief- MERL researchers are presenting 5 conference papers, 3 workshop papers, and are co-organizing two workshops at the CVPR 2024 conference, which will be held in Seattle, June 17-21. CVPR is one of the most prestigious and competitive international conferences in computer vision. Details of MERL contributions are provided below.
CVPR Conference Papers:
1. "TI2V-Zero: Zero-Shot Image Conditioning for Text-to-Video Diffusion Models" by H. Ni, B. Egger, S. Lohit, A. Cherian, Y. Wang, T. Koike-Akino, S. X. Huang, and T. K. Marks
This work enables a pretrained text-to-video (T2V) diffusion model to be additionally conditioned on an input image (first video frame), yielding a text+image to video (TI2V) model. Other than using the pretrained T2V model, our method requires no ("zero") training or fine-tuning. The paper uses a "repeat-and-slide" method and diffusion resampling to synthesize videos from a given starting image and text describing the video content.
Paper: https://www.merl.com/publications/TR2024-059
Project page: https://merl.com/research/highlights/TI2V-Zero
2. "Long-Tailed Anomaly Detection with Learnable Class Names" by C.-H. Ho, K.-C. Peng, and N. Vasconcelos
This work aims to identify defects across various classes without relying on hard-coded class names. We introduce the concept of long-tailed anomaly detection, addressing challenges like class imbalance and dataset variability. Our proposed method combines reconstruction and semantic modules, learning pseudo-class names and utilizing a variational autoencoder for feature synthesis to improve performance in long-tailed datasets, outperforming existing methods in experiments.
Paper: https://www.merl.com/publications/TR2024-040
3. "Gear-NeRF: Free-Viewpoint Rendering and Tracking with Motion-aware Spatio-Temporal Sampling" by X. Liu, Y-W. Tai, C-T. Tang, P. Miraldo, S. Lohit, and M. Chatterjee
This work presents a new strategy for rendering dynamic scenes from novel viewpoints. Our approach is based on stratifying the scene into regions based on the extent of motion of the region, which is automatically determined. Regions with higher motion are permitted a denser spatio-temporal sampling strategy for more faithful rendering of the scene. Additionally, to the best of our knowledge, ours is the first work to enable tracking of objects in the scene from novel views - based on the preferences of a user, provided by a click.
Paper: https://www.merl.com/publications/TR2024-042
4. "SIRA: Scalable Inter-frame Relation and Association for Radar Perception" by R. Yataka, P. Wang, P. T. Boufounos, and R. Takahashi
Overcoming the limitations on radar feature extraction such as low spatial resolution, multipath reflection, and motion blurs, this paper proposes SIRA (Scalable Inter-frame Relation and Association) for scalable radar perception with two designs: 1) extended temporal relation, generalizing the existing temporal relation layer from two frames to multiple inter-frames with temporally regrouped window attention for scalability; and 2) motion consistency track with a pseudo-tracklet generated from observational data for better object association.
Paper: https://www.merl.com/publications/TR2024-041
5. "RILA: Reflective and Imaginative Language Agent for Zero-Shot Semantic Audio-Visual Navigation" by Z. Yang, J. Liu, P. Chen, A. Cherian, T. K. Marks, J. L. Roux, and C. Gan
We leverage Large Language Models (LLM) for zero-shot semantic audio visual navigation. Specifically, by employing multi-modal models to process sensory data, we instruct an LLM-based planner to actively explore the environment by adaptively evaluating and dismissing inaccurate perceptual descriptions.
Paper: https://www.merl.com/publications/TR2024-043
CVPR Workshop Papers:
1. "CoLa-SDF: Controllable Latent StyleSDF for Disentangled 3D Face Generation" by R. Dey, B. Egger, V. Boddeti, Y. Wang, and T. K. Marks
This paper proposes a new method for generating 3D faces and rendering them to images by combining the controllability of nonlinear 3DMMs with the high fidelity of implicit 3D GANs. Inspired by StyleSDF, our model uses a similar architecture but enforces the latent space to match the interpretable and physical parameters of the nonlinear 3D morphable model MOST-GAN.
Paper: https://www.merl.com/publications/TR2024-045
2. “Tracklet-based Explainable Video Anomaly Localization” by A. Singh, M. J. Jones, and E. Learned-Miller
This paper describes a new method for localizing anomalous activity in video of a scene given sample videos of normal activity from the same scene. The method is based on detecting and tracking objects in the scene and estimating high-level attributes of the objects such as their location, size, short-term trajectory and object class. These high-level attributes can then be used to detect unusual activity as well as to provide a human-understandable explanation for what is unusual about the activity.
Paper: https://www.merl.com/publications/TR2024-057
MERL co-organized workshops:
1. "Multimodal Algorithmic Reasoning Workshop" by A. Cherian, K-C. Peng, S. Lohit, M. Chatterjee, H. Zhou, K. Smith, T. K. Marks, J. Mathissen, and J. Tenenbaum
Workshop link: https://marworkshop.github.io/cvpr24/index.html
2. "The 5th Workshop on Fair, Data-Efficient, and Trusted Computer Vision" by K-C. Peng, et al.
Workshop link: https://fadetrcv.github.io/2024/
3. "SuperLoRA: Parameter-Efficient Unified Adaptation for Large Vision Models" by X. Chen, J. Liu, Y. Wang, P. Wang, M. Brand, G. Wang, and T. Koike-Akino
This paper proposes a generalized framework called SuperLoRA that unifies and extends different variants of low-rank adaptation (LoRA). Introducing new options with grouping, folding, shuffling, projection, and tensor decomposition, SuperLoRA offers high flexibility and demonstrates superior performance up to 10-fold gain in parameter efficiency for transfer learning tasks.
Paper: https://www.merl.com/publications/TR2024-062
- MERL researchers are presenting 5 conference papers, 3 workshop papers, and are co-organizing two workshops at the CVPR 2024 conference, which will be held in Seattle, June 17-21. CVPR is one of the most prestigious and competitive international conferences in computer vision. Details of MERL contributions are provided below.
-
NEWS MERL researchers presenting four papers and organizing the VLAR-SMART101 Workshop at ICCV 2023 Date: October 2, 2023 - October 6, 2023
Where: Paris/France
MERL Contacts: Moitreya Chatterjee; Anoop Cherian; Michael J. Jones; Toshiaki Koike-Akino; Suhas Lohit; Tim K. Marks; Pedro Miraldo; Kuan-Chuan Peng; Ye Wang
Research Areas: Artificial Intelligence, Computer Vision, Machine LearningBrief- MERL researchers are presenting 4 papers and organizing the VLAR-SMART-101 workshop at the ICCV 2023 conference, which will be held in Paris, France October 2-6. ICCV is one of the most prestigious and competitive international conferences in computer vision. Details are provided below.
1. Conference paper: “Steered Diffusion: A Generalized Framework for Plug-and-Play Conditional Image Synthesis,” by Nithin Gopalakrishnan Nair, Anoop Cherian, Suhas Lohit, Ye Wang, Toshiaki Koike-Akino, Vishal Patel, and Tim K. Marks
Conditional generative models typically demand large annotated training sets to achieve high-quality synthesis. As a result, there has been significant interest in plug-and-play generation, i.e., using a pre-defined model to guide the generative process. In this paper, we introduce Steered Diffusion, a generalized framework for fine-grained photorealistic zero-shot conditional image generation using a diffusion model trained for unconditional generation. The key idea is to steer the image generation of the diffusion model during inference via designing a loss using a pre-trained inverse model that characterizes the conditional task. Our model shows clear qualitative and quantitative improvements over state-of-the-art diffusion-based plug-and-play models, while adding negligible computational cost.
2. Conference paper: "BANSAC: A dynamic BAyesian Network for adaptive SAmple Consensus," by Valter Piedade and Pedro Miraldo
We derive a dynamic Bayesian network that updates individual data points' inlier scores while iterating RANSAC. At each iteration, we apply weighted sampling using the updated scores. Our method works with or without prior data point scorings. In addition, we use the updated inlier/outlier scoring for deriving a new stopping criterion for the RANSAC loop. Our method outperforms the baselines in accuracy while needing less computational time.
3. Conference paper: "Robust Frame-to-Frame Camera Rotation Estimation in Crowded Scenes," by Fabien Delattre, David Dirnfeld, Phat Nguyen, Stephen Scarano, Michael J. Jones, Pedro Miraldo, and Erik Learned-Miller
We present a novel approach to estimating camera rotation in crowded, real-world scenes captured using a handheld monocular video camera. Our method uses a novel generalization of the Hough transform on SO3 to efficiently find the camera rotation most compatible with the optical flow. Because the setting is not addressed well by other data sets, we provide a new dataset and benchmark, with high-accuracy and rigorously annotated ground truth on 17 video sequences. Our method is more accurate by almost 40 percent than the next best method.
4. Workshop paper: "Tensor Factorization for Leveraging Cross-Modal Knowledge in Data-Constrained Infrared Object Detection" by Manish Sharma*, Moitreya Chatterjee*, Kuan-Chuan Peng, Suhas Lohit, and Michael Jones
While state-of-the-art object detection methods for RGB images have reached some level of maturity, the same is not true for Infrared (IR) images. The primary bottleneck towards bridging this gap is the lack of sufficient labeled training data in the IR images. Towards addressing this issue, we present TensorFact, a novel tensor decomposition method which splits the convolution kernels of a CNN into low-rank factor matrices with fewer parameters. This compressed network is first pre-trained on RGB images and then augmented with only a few parameters. This augmented network is then trained on IR images, while freezing the weights trained on RGB. This prevents it from over-fitting, allowing it to generalize better. Experiments show that our method outperforms state-of-the-art.
5. “Vision-and-Language Algorithmic Reasoning (VLAR) Workshop and SMART-101 Challenge” by Anoop Cherian, Kuan-Chuan Peng, Suhas Lohit, Tim K. Marks, Ram Ramrakhya, Honglu Zhou, Kevin A. Smith, Joanna Matthiesen, and Joshua B. Tenenbaum
MERL researchers along with researchers from MIT, GeorgiaTech, Math Kangaroo USA, and Rutgers University are jointly organizing a workshop on vision-and-language algorithmic reasoning at ICCV 2023 and conducting a challenge based on the SMART-101 puzzles described in the paper: Are Deep Neural Networks SMARTer than Second Graders?. A focus of this workshop is to bring together outstanding faculty/researchers working at the intersections of vision, language, and cognition to provide their opinions on the recent breakthroughs in large language models and artificial general intelligence, as well as showcase their cutting edge research that could inspire the audience to search for the missing pieces in our quest towards solving the puzzle of artificial intelligence.
Workshop link: https://wvlar.github.io/iccv23/
- MERL researchers are presenting 4 papers and organizing the VLAR-SMART-101 workshop at the ICCV 2023 conference, which will be held in Paris, France October 2-6. ICCV is one of the most prestigious and competitive international conferences in computer vision. Details are provided below.
See All News & Events for Mike -
-
Awards
-
AWARD CVPR 2011 Longuet-Higgins Prize Date: June 25, 2011
Awarded to: Paul A. Viola and Michael J. Jones
Awarded for: "Rapid Object Detection using a Boosted Cascade of Simple Features"
Awarded by: Conference on Computer Vision and Pattern Recognition (CVPR)
MERL Contact: Michael J. Jones
Research Area: Machine LearningBrief- Paper from 10 years ago with the largest impact on the field: "Rapid Object Detection using a Boosted Cascade of Simple Features", originally published at Conference on Computer Vision and Pattern Recognition (CVPR 2001).
-
-
Research Highlights
-
Internships with Mike
-
CV0060: Internship - Video Anomaly Detection
MERL is looking for a self-motivated intern to work on the problem of video anomaly detection. The intern will help to develop new ideas for improving the state of the art in detecting anomalous activity in videos. The ideal candidate would be a Ph.D. student with a strong background in machine learning and computer vision and some experience with video anomaly detection in particular. Proficiency in Python programming and Pytorch is necessary. The successful candidate is expected to have published at least one paper in a top-tier computer vision or machine learning venue, such as CVPR, ECCV, ICCV, WACV, ICML, ICLR, NeurIPS or AAAI. The intern will collaborate with MERL researchers to develop and test algorithms and prepare manuscripts for scientific publications. The internship is for 3 months and the start date is flexible.
Required Specific Experience
- Graduate student in Ph.D. program
- Experience with PyTorch.
- Prior publication in computer vision or machine learning conference/journal.
-
-
MERL Publications
- "ComplexVAD: Detecting Interaction Anomalies in Video", IEEE Winter Conference on Applications of Computer Vision (WACV) Workshop, February 2025.BibTeX TR2025-016 PDF
- @inproceedings{Mumcu2025feb,
- author = {Mumcu, Furkan and Jones, Michael J. and Yilmaz, Yasin and Cherian, Anoop},
- title = {{ComplexVAD: Detecting Interaction Anomalies in Video}},
- booktitle = {IEEE Winter Conference on Applications of Computer Vision (WACV) Workshop},
- year = 2025,
- month = feb,
- url = {https://www.merl.com/publications/TR2025-016}
- }
, - "Equivariant Spatio-Temporal Self-Supervision for LiDAR Object Detection", European Conference on Computer Vision (ECCV), Leonardis, A. and Ricci, E. and Roth, S. and Russakovsky, O. and Sattler, T. and Varol, G., Eds., DOI: 10.1007/978-3-031-73347-5_27, September 2024, pp. 475-491.BibTeX TR2024-130 PDF Video Presentation
- @inproceedings{Hegde2024sep,
- author = {Hegde, Deepti and Lohit, Suhas and Peng, Kuan-Chuan and Jones, Michael J. and Patel, Vishal M.},
- title = {{Equivariant Spatio-Temporal Self-Supervision for LiDAR Object Detection}},
- booktitle = {European Conference on Computer Vision (ECCV)},
- year = 2024,
- editor = {Leonardis, A. and Ricci, E. and Roth, S. and Russakovsky, O. and Sattler, T. and Varol, G.},
- pages = {475--491},
- month = sep,
- publisher = {Springer},
- doi = {10.1007/978-3-031-73347-5_27},
- issn = {0302-9743},
- isbn = {978-3-031-73346-8},
- url = {https://www.merl.com/publications/TR2024-130}
- }
, - "Tracklet-based Explainable Video Anomaly Localization", IEEE Conference on Computer Vision and Pattern Recognition (CVPR) Workshops, May 2024, pp. 3992-4001.BibTeX TR2024-057 PDF
- @inproceedings{Singh2024may,
- author = {Singh, Ashish and Jones, Michael J. and Learned-Miller, Erik},
- title = {{Tracklet-based Explainable Video Anomaly Localization}},
- booktitle = {IEEE Conference on Computer Vision and Pattern Recognition (CVPR) Workshops},
- year = 2024,
- pages = {3992--4001},
- month = may,
- url = {https://www.merl.com/publications/TR2024-057}
- }
, - "Multimodal 3D Object Detection on Unseen Domains", arXiv, April 2024. ,
- "Pixel-Grounded Prototypical Part Networks", IEEE Winter Conference on Applications of Computer Vision (WACV), DOI: 10.1109/WACV57701.2024.00470, January 2024.BibTeX TR2024-002 PDF Video Software Presentation
- @inproceedings{Carmichael2024jan,
- author = {Carmichael, Zachariah and Jones, Lohit, Suhas and Cherian, Anoop and Michael J. and Scheirer, Walter},
- title = {{Pixel-Grounded Prototypical Part Networks}},
- booktitle = {IEEE Winter Conference on Applications of Computer Vision (WACV)},
- year = 2024,
- month = jan,
- doi = {10.1109/WACV57701.2024.00470},
- url = {https://www.merl.com/publications/TR2024-002}
- }
,
- "ComplexVAD: Detecting Interaction Anomalies in Video", IEEE Winter Conference on Applications of Computer Vision (WACV) Workshop, February 2025.
-
Other Publications
- "Lfw results using a combined nowak plus merl recognizer", 2008.BibTeX
- @Article{huang2008lfw,
- author = {Huang, G.B. and Jones, M.J. and Learned-Miller, E. and others},
- title = {Lfw results using a combined nowak plus merl recognizer},
- year = 2008
- }
, - "Analogous view transfer for gaze correction in video sequences", Control, Automation, Robotics and Vision, 2002. ICARCV 2002. 7th International Conference on, 2002, vol. 3, pp. 1415-1420.BibTeX
- @Inproceedings{cham2002analogous,
- author = {Cham, T.J. and Krishnamoorthy, S. and Jones, M.},
- title = {Analogous view transfer for gaze correction in video sequences},
- booktitle = {Control, Automation, Robotics and Vision, 2002. ICARCV 2002. 7th International Conference on},
- year = 2002,
- volume = 3,
- pages = {1415--1420},
- organization = {IEEE}
- }
, - "Statistical color models with application to skin detection", International Journal of Computer Vision, Vol. 46, No. 1, pp. 81-96, 2002.BibTeX
- @Article{jones2002statistical,
- author = {Jones, M.J. and Rehg, J.M.},
- title = {Statistical color models with application to skin detection},
- journal = {International Journal of Computer Vision},
- year = 2002,
- volume = 46,
- number = 1,
- pages = {81--96},
- publisher = {Springer}
- }
, - "Appearance-based structure from motion using linear classes of 3-d models", International Journal of Computer Vision, Vol. 49, No. 1, pp. 5-22, 2002.BibTeX
- @Article{kang2002appearance,
- author = {Kang, S.B. and Jones, M.},
- title = {Appearance-based structure from motion using linear classes of 3-d models},
- journal = {International Journal of Computer Vision},
- year = 2002,
- volume = 49,
- number = 1,
- pages = {5--22},
- publisher = {Springer}
- }
, - "A Monte Carlo algorithm for fast projective clustering", Proceedings of the 2002 ACM SIGMOD international conference on Management of data, 2002, pp. 418-427.BibTeX
- @Inproceedings{procopiuc2002monte,
- author = {Procopiuc, C.M. and Jones, M. and Agarwal, P.K. and Murali, TM},
- title = {A Monte Carlo algorithm for fast projective clustering},
- booktitle = {Proceedings of the 2002 ACM SIGMOD international conference on Management of data},
- year = 2002,
- pages = {418--427},
- organization = {ACM}
- }
, - "Fast and robust classification using asymmetric adaboost and a detector cascade", Proc. of NIPS01, 2001.BibTeX
- @Article{viola2001fast,
- author = {Viola, P. and Jones, M.},
- title = {Fast and robust classification using asymmetric adaboost and a detector cascade},
- journal = {Proc. of NIPS01},
- year = 2001
- }
, - "Statistical color models with application to skin detection", Computer Vision and Pattern Recognition, 1999. IEEE Computer Society Conference on., 1999, vol. 1.BibTeX
- @Inproceedings{jones1999statistical,
- author = {Jones, M.J. and Rehg, J.M.},
- title = {Statistical color models with application to skin detection},
- booktitle = {Computer Vision and Pattern Recognition, 1999. IEEE Computer Society Conference on.},
- year = 1999,
- volume = 1,
- organization = {IEEE}
- }
, - "A cluster-based statistical model for object detection", Computer Vision, 1999. The Proceedings of the Seventh IEEE International Conference on, 1999, vol. 2, pp. 1046-1053.BibTeX
- @Inproceedings{rikert1999cluster,
- author = {Rikert, T.D. and Jones, M.J. and Viola, P.},
- title = {A cluster-based statistical model for object detection},
- booktitle = {Computer Vision, 1999. The Proceedings of the Seventh IEEE International Conference on},
- year = 1999,
- volume = 2,
- pages = {1046--1053},
- organization = {IEEE}
- }
, - "Hierarchical morphable models", Computer Vision and Pattern Recognition, 1998. Proceedings. 1998 IEEE Computer Society Conference on, 1998, pp. 820-826.BibTeX
- @Inproceedings{jones1998hierarchical,
- author = {Jones, M.J. and Poggio, T.},
- title = {Hierarchical morphable models},
- booktitle = {Computer Vision and Pattern Recognition, 1998. Proceedings. 1998 IEEE Computer Society Conference on},
- year = 1998,
- pages = {820--826},
- organization = {IEEE}
- }
, - "Multidimensional morphable models: A framework for representing and matching object classes", International Journal of Computer Vision, Vol. 29, No. 2, pp. 107-131, 1998.BibTeX
- @Article{jones1998multidimensional,
- author = {Jones, M.J. and Poggio, T.},
- title = {Multidimensional morphable models: A framework for representing and matching object classes},
- journal = {International Journal of Computer Vision},
- year = 1998,
- volume = 29,
- number = 2,
- pages = {107--131},
- publisher = {Springer}
- }
, - "Gaze estimation using morphable models", Automatic Face and Gesture Recognition, 1998. Proceedings. Third IEEE International Conference on, 1998, pp. 436-441.BibTeX
- @Inproceedings{rikert1998gaze,
- author = {Rikert, T.D. and Jones, M.J.},
- title = {Gaze estimation using morphable models},
- booktitle = {Automatic Face and Gesture Recognition, 1998. Proceedings. Third IEEE International Conference on},
- year = 1998,
- pages = {436--441},
- organization = {IEEE}
- }
, - "Multidimensional morphable models: A framework for representing and matching object classes", 1997.BibTeX
- @Phdthesis{jones1997multidimensional,
- author = {Jones, M.J.},
- title = {Multidimensional morphable models: A framework for representing and matching object classes},
- year = 1997,
- publisher = {Massachusetts Institute of Technology}
- }
, - "Top-down learning of low-level vision tasks", Current Biology, Vol. 7, No. 12, pp. 991-994, 1997.BibTeX
- @Article{jones1997top,
- author = {Jones, M.J. and Sinha, P. and Vetter, T. and Poggio, T.},
- title = {Top-down learning of low-level vision tasks},
- journal = {Current Biology},
- year = 1997,
- volume = 7,
- number = 12,
- pages = {991--994},
- publisher = {Elsevier}
- }
, - "A bootstrapping algorithm for learning linear models of object classes", Computer Vision and Pattern Recognition, 1997. Proceedings., 1997 IEEE Computer Society Conference on, 1997, pp. 40-46.BibTeX
- @Inproceedings{vetter1997bootstrapping,
- author = {Vetter, T. and Jones, M.J. and Poggio, T.},
- title = {A bootstrapping algorithm for learning linear models of object classes},
- booktitle = {Computer Vision and Pattern Recognition, 1997. Proceedings., 1997 IEEE Computer Society Conference on},
- year = 1997,
- pages = {40--46},
- organization = {IEEE}
- }
, - "Regularization theory and neural networks architectures", Neural computation, Vol. 7, No. 2, pp. 219-269, 1995.BibTeX
- @Article{girosi1995regularization,
- author = {Girosi, F. and Jones, M. and Poggio, T.},
- title = {Regularization theory and neural networks architectures},
- journal = {Neural computation},
- year = 1995,
- volume = 7,
- number = 2,
- pages = {219--269},
- publisher = {MIT Press}
- }
, - "Model-based matching of line drawings by linear combinations of prototypes", Computer Vision, 1995. Proceedings., Fifth International Conference on, 1995, pp. 531-536.BibTeX
- @Inproceedings{jones1995model,
- author = {Jones, M.J. and Poggio, T.},
- title = {Model-based matching of line drawings by linear combinations of prototypes},
- booktitle = {Computer Vision, 1995. Proceedings., Fifth International Conference on},
- year = 1995,
- pages = {531--536},
- organization = {IEEE}
- }
, - "Priors stabilizers and basis functions: From regularization to radial, tensor and additive splines", MIT AI Lab Memo 1430, 1993.BibTeX
- @Article{girosi1993priors,
- author = {Girosi, F. and Jones, M. and Poggio, T.},
- title = {Priors stabilizers and basis functions: From regularization to radial, tensor and additive splines},
- journal = {MIT AI Lab Memo 1430},
- year = 1993
- }
, - "From regularization to radial, tensor and additive splines", Neural Networks, 1993. IJCNN'93-Nagoya. Proceedings of 1993 International Joint Conference on, 1993, vol. 1, pp. 223-227.BibTeX
- @Inproceedings{poggio1993regularization,
- author = {Poggio, T. and Girosi, F. and Jones, M.},
- title = {From regularization to radial, tensor and additive splines},
- booktitle = {Neural Networks, 1993. IJCNN'93-Nagoya. Proceedings of 1993 International Joint Conference on},
- year = 1993,
- volume = 1,
- pages = {223--227},
- organization = {IEEE}
- }
, - "Using recurrent networks for dimensionality reduction", 1992, Massachusetts Institute of Technology.BibTeX
- @Mastersthesis{jones1992using,
- author = {Jones, M.J.},
- title = {Using recurrent networks for dimensionality reduction},
- school = {Massachusetts Institute of Technology},
- year = 1992
- }
,
- "Lfw results using a combined nowak plus merl recognizer", 2008.
-
Software & Data Downloads
-
ComplexVAD Dataset -
Pixel-Grounded Prototypical Part Networks -
Robust Frame-to-Frame Camera Rotation Estimation in Crowded Scenes
-
Explainable Video Anomaly Localization -
Street Scene Dataset -
Landmarks’ Location, Uncertainty, and Visibility Likelihood -
MERL Shopping Dataset -
Exemplar-Based Anomaly Detection
-
-
Videos
-
MERL Issued Patents
-
Title: "System and Method for Anomaly Detection of a Scene"
Inventors: Jones, Michael J.
Patent No.: 12,106,562
Issue Date: Oct 1, 2024 -
Title: "System and Method for Detecting Objects in Video Sequences"
Inventors: Jones, Michael J.; Broad, Alexander
Patent No.: 11,164,003
Issue Date: Nov 2, 2021 -
Title: "System and Method for Detecting Motion Anomalies in Video"
Inventors: Jones, Michael J.
Patent No.: 10,970,823
Issue Date: Apr 6, 2021 -
Title: "System and Method for Detecting Anomalies in Video using a Similarity Function Trained by Machine Learning"
Inventors: Jones, Michael J.
Patent No.: 10,824,935
Issue Date: Nov 3, 2020 -
Title: "Method and System for Determining 3D Object Poses and Landmark Points using Surface Patches"
Inventors: Jones, Michael J.; Marks, Tim; Papazov, Chavdar
Patent No.: 10,515,259
Issue Date: Dec 24, 2019 -
Title: "System and Method for Image Comparison Based on Hyperplanes Similarity"
Inventors: Jones, Michael J.
Patent No.: 10,452,958
Issue Date: Oct 22, 2019 -
Title: "Method and System for Detecting Actions in Videos"
Inventors: Jones, Michael J.; Marks, Tim; Tuzel, Oncel; Singh, Bharat
Patent No.: 10,242,266
Issue Date: Mar 26, 2019 -
Title: "Method and System for Detecting Actions in Videos using Contour Sequences"
Inventors: Jones, Michael J.; Marks, Tim; Kulkarni, Kuldeep
Patent No.: 10,210,391
Issue Date: Feb 19, 2019 -
Title: "Method for Anomaly Detection in Time Series Data Based on Spectral Partitioning"
Inventors: Nikovski, Daniel N.; Kniazev, Andrei; Jones, Michael J.
Patent No.: 9,984,334
Issue Date: May 29, 2018 -
Title: "Method for Learning Exemplars for Anomaly Detection"
Inventors: Jones, Michael J.; Nikovski, Daniel N.
Patent No.: 9,779,361
Issue Date: Oct 3, 2017 -
Title: "Method for Determining Similarity of Objects Represented in Images"
Inventors: Jones, Michael J.; Marks, Tim; Ahmed, Ejaz
Patent No.: 9,436,895
Issue Date: Sep 6, 2016 -
Title: "Method and System for Tracking People in Indoor Environments using a Visible Light Camera and a Low-Frame-Rate Infrared Sensor"
Inventors: Marks, Tim; Jones, Michael J.; Kumar, Suren
Patent No.: 9,245,196
Issue Date: Jan 26, 2016 -
Title: "Method for Detecting and Tracking Objects in Image Sequences of Scenes Acquired by a Stationary Camera"
Inventors: Marks, Tim; Jones, Michael J.; MV, Rohith
Patent No.: 9,213,896
Issue Date: Dec 15, 2015 -
Title: "Method for Detecting Anomalies in a Time Series Data with Trajectory and Stochastic Components"
Inventors: Jones, Michael J.
Patent No.: 9,146,800
Issue Date: Sep 29, 2015 -
Title: "Method for Predicting Future Travel Time Using Geospatial Inference"
Inventors: Jones, Michael J.; Nikovski, Daniel N.; Geng, Yanfeng
Patent No.: 9,122,987
Issue Date: Sep 1, 2015 -
Title: "Method for Detecting Anomalies in Multivariate Time Series Data"
Inventors: Nikovski, Daniel N.; Jones, Michael J.
Patent No.: 9,075,713
Issue Date: Jul 7, 2015 -
Title: "Camera-Based 3D Climate Control"
Inventors: Marks, Tim; Jones, Michael J.
Patent No.: 8,929,592
Issue Date: Jan 6, 2015 -
Title: "Object Detection in Depth Images"
Inventors: Jones, Michael J.; Tuzel, C. Oncel; Si, Weiguang
Patent No.: 8,406,470
Issue Date: Mar 26, 2013 -
Title: "Method for identifying Faces in Images with Improved Accuracy Using Compressed Feature Vectors"
Inventors: Thornton, Jay E.; Jones, Michael J.
Patent No.: 8,213,691
Issue Date: Jul 3, 2012 -
Title: "Method for Synthetically Images of Objects"
Inventors: Jones, Michael J.; Marks, Tim; Kumar, Ritwik
Patent No.: 8,194,072
Issue Date: Jun 5, 2012 -
Title: "Method for Localizing Irises in Images Using Gradients and Textures"
Inventors: Jones, Michael J.; Guo, Guo Dong
Patent No.: 7,583,823
Issue Date: Sep 1, 2009 -
Title: "Detecting Pedestrians Using Patterns of Motion and Appearance in Videos"
Inventors: Jones, Michael J.; Viola, Paul A.
Patent No.: 7,212,651
Issue Date: May 1, 2007 -
Title: "Detecting Arbitrarily Oriented Objects in Images"
Inventors: Viola, Paul A.; Jones, Michael J.
Patent No.: 7,197,186
Issue Date: Mar 27, 2007 -
Title: "Object Recognition System"
Inventors: Viola, Paul A.; Jones, Michael J.
Patent No.: 7,031,499
Issue Date: Apr 18, 2006 -
Title: "System and Method for Detecting Objects in Images"
Inventors: Viola, Paul A.; Jones, Michael J.
Patent No.: 7,020,337
Issue Date: Mar 28, 2006
-
Title: "System and Method for Anomaly Detection of a Scene"